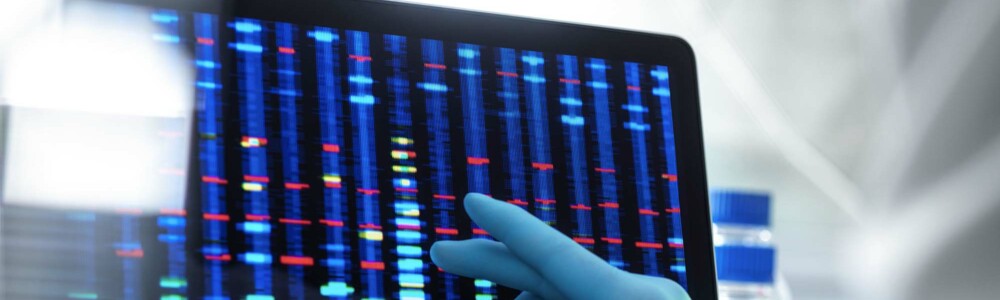
The impact of algorithms and big data on drug discovery and development processes: An empirical study
Computational science refers to the design and use of artificial intelligence tools and computer simulations and it is becoming established across an ever-wider spectrum of the drug discovery and development process. Biotech companies are leading the charge. Drug makers are expecting the widespread development of AI algorithms and big data platforms to benefit their own drug research and development activities in many ways. Connecting and evaluating data using AI will save them both time and money during the preclinical and clinical phases of drug development. Drug makers are also seeking to steadily reduce the risk of clinical failure thanks to the increasing accuracy and precision of their data analysis capabilities. Last but not least, AI-optimized drug discovery processes are being used to identify clinical targets with molecular structures that offer a clearly superior mechanism of action in a wide range of therapeutic areas.
Many drug developers are developing their own algorithms and big data platforms to leverage their in-house clinical and scientific data and setting up new hardware systems, as they seek to enhance their R&D pipelines with AI and big-data computing capabilities. In pursuit of these objectives, they are, on the one hand, deploying machine learning tools in data analysis and, on the other hand, conducting computer simulations in the design, development, and clinical testing of new drugs. In practical terms, the technologies are being used throughout the various phases of preclinical and clinical R&D – from target selection and the identification and optimization of lead compounds to the selection of patients for a clinical trial.
A study analyzing AI and drug discovery
BB Biotech recognized early on that automated algorithms can be used within the scope of its own investment process to analyze the growing mountain of data generated by the biopharmaceutical industry more efficiently. In an internal project called BioCarta managed by three data scientists on the company’s payroll, it created a proprietary data pool along with the necessary analytics software, big data apps, and hardware.
BB Biotech’s Investment Management team and external experts conducted a study to obtain an overview of how and to what extent computational science can improve future drug discovery and development processes. A total of 90 companies that claim that computational science is a crucial component of their operating business and that also met certain criteria specified by BB Biotech were covered in this study.
Their business models, strategies and funding profiles were examined in the study. There were some major differences between the business models. More than half of the 90 companies are services providers. The remaining 41% of the companies are partnering with pharmaceutical companies in the development of up to 49% of their pipeline projects. In effect, this means that only 21% of the companies studied are applying the latest computer science technology in the development of their pipeline projects and financing these activities on their own.
Focus on algorithms and early-stage research
93% of the companies surveyed say that the new algorithms they are using give them an advantage over the competition in the drug discovery and development process. However, only 6.5% of the companies say that the hardware they use gives them a competitive advantage. This discrepancy can largely be traced to cost factors. New algorithms can be developed at relatively low cost, whereas it takes considerably more capital to buy the necessary hardware. This hardware is mainly used for drug design and target identification purposes, primarily to predict molecular drug-target interactions. That information is then used to draw conclusions about molecular interaction and binding affinity. Relay Therapeutics, a biotech company in which BB Biotech is invested, and Modulus Discovery are using this approach in their drug development pipelines.
37% of the companies surveyed say that the integration of AI-based data sets into their R&D pipeline has advanced their biological and clinical understanding of a targeted disease or condition. Most of the companies that have integrated AI-based data into their pipeline are still in the early stages of clinical development, except for a few exceptions (such as Recursion).
Only 28% of the drug companies analyzed in the study have proprietary products at an advanced stage of clinical development with proven efficacy. Computational science technology is being used most often to determine profiles for clinical trials and for clinical trial participants. AI still plays a minor role in determining active ingredients and managing safety aspects: A mere 2.2% and 4.1%, respectively, of the companies surveyed use computer-based data for these purposes. The physiological complexity of the molecules is considered one of the greatest challenges when conducting in silico studies (drug R&D conducted exclusively on a computer or via computer simulation), along with the high cost of in vivo testing, i.e. drug R&D carried out with or in living organisms. In silico-based toxicological studies in novel therapeutic applications are still not widely accepted by regulators. That could change in the US, where the FDA is endeavoring to ease the regulations for in vivo studies, which could lead to the greater standardization of in silico protocols. This would lead to greater recognition of these protocols in regulatory processes.
More partnering deals and IPOs
An analysis of the funding profiles of companies with a self-developed or partnered R&D pipeline showed that private equity vehicles and equity funds have provided the most capital to early-phase companies using their own algorithms. Companies that are using their own AI tools, either for preclinical drug discovery purposes or in early phase clinical trials, have raised an average of USD 237 mn in outside funding. The average amount of funding provided to companies developing algorithms for analyzing patient data is slightly higher at USD 300 mn. The picture is quite different at companies that are focused on capital-intensive hardware: They have raised an average of USD 622 mn.
Judging by the increasing number of IPOs over the past three years and the generally growing investment inflows, the segment of biotech companies actively applying computational science is maturing and developing. Partnership deals involving the pharmaceutical industry have clearly been focused on services, which is reflected in the large number of companies in the study whose business models center on services and not on drug discovery and development. Looking ahead, it will be exciting to see if and how the number of collaboration agreements in which computer-based technology is integrated into late-stage clinical development projects increases. A key argument here will be the potential cost savings in pivotal clinical trials: The bulk of a drug's total R&D cost is incurred during this phase of drug development.
Commercial added value
Commercial added value is a key question that was raised already during the planning process for the study: How will computational science generate value with respect to new drug approvals, both in terms of their number and the therapeutic benefit of the approved drugs? Clear conclusions cannot yet be drawn here. 71% of all pipeline projects are still in a preclinical phase of development. The modalities currently in Phase I or II clinical trials will be crucial in determining whether AI and big data will help companies to bring more effective and cheaper drugs to the market going forward. Currently, most of the companies covered by the study are focused on algorithms and target validation, primarily in the two areas of oncology and neurological disorders. In view of the molecular structures of therapeutic antibodies and peptides, and the growing number of regulatory approvals, it is conceivable that AI could help to lower the high manufacturing costs associated with these drugs. At present, small molecule drugs still account for 61% of all ongoing R&D projects in which AI-based technology is being applied.
Therefore, it is too early to draw any specific conclusions comparing the success of computational science in drug R&D projects with the success rates of drug R&D projects that use other technologies. The growing number of collaboration agreements is a clear sign that computational drug research and development has attained a new level of maturity. The developments and outcomes of the coming years will show to what extent computational science can accelerate the development and commercialization of innovative drugs. BB Biotech’s Investment Management Team will continue to closely monitor sector developments, engaging early on with companies pursuing novel approaches and platform technologies, and ultimately make its investment decisions based on specific arguments rather than, say, on the vague potential of a certain platform.
Three specialists in BB Biotech’s investment portfolio
BB Biotech is currently invested in three biotech companies that have made computational science an important factor in their research and development activities.